As recently as the late 1990s and early 2000s--speaking anecdotally--catching a blockbuster at the cinema routinely involved evaluating its “special effects” afterwards. It seemed that each year brought the realism of exploding spaceships and angry dinosaurs to dazzling new heights.
At a certain point, however, evaluations of this type died out among the casual viewer, ostensibly because “lifelike” is a superlative concept. Once verisimilitude is complete, it cannot be augmented. The spaceships are exploding. The dinosaurs are angry.
But while cinematic artists may remain at the forefront of special effects, the field is no longer exclusive. Software powered by deep learning (a type of artificial intelligence) has brought us deepfakes, i.e. images or videos that look real but are not. Deepfakes are cheap and easy to make, and in the wrong hands pose several dangers: scammers can ask for money while posing as someone trustworthy, people can be non-consensually edited into pornographic videos, voters can be misled with false images or films, etc.
Cat and mouse, but mostly mouse
In 2019, that for every 100 people working on synthesising deepfake videos, only one was working on how to detect them.
A team of researchers at the SnT--the Interdisciplinary Centre for Security, Reliability and Trust at the University of Luxembourg--have joined the fight, however. Headed by senior research scientist Djamila Aouada, the team’s three-year project officially started on 1 March with the aim of developing a new method for detecting deepfakes. The project has been dubbed “DeepFake Detection using Spatio-Temporal Spectral Representations for Effective Learning”, aka FakeDeTer.
Currently, Aouada explains, deepfake detection methods are tailored to specific deepfake generators. The fake videos are made using generative adversarial networks (GANs), or deep learning models where two neural networks compete and respectively try to become more accurate than the other; every GAN uses a variation on the model, a signature pattern that is specifically targeted by detection tools.
But this approach is a losing game, since the videomakers outnumber the detectors so badly. “Obviously, they’re winning the race,” Aouada comments. “Which is why we’re changing strategy.”
The SnT team’s strategy is thus to create a tool that isn’t tied to any specific GAN. In other words, a generic approach where it doesn’t matter how the deepfake was generated, where the goal is merely to determine what is unlikely to be real. “And how you do that is by defining the normal,” says Aouada, adding that there is a movement within the detection community towards this type of approach.
The project is focused particularly on face-swaps, a common type of deepfake. “We have a strong a priori knowledge about what a face is like, how it moves, etc.,” the researcher explains. “So we use that to define a normal representation. And anything that deviates in one way or another--this is what we want to detect.”
To that end, the project involves 3D-face modelling, an area already familiar to the research team. They’re looking at patterns specific to the face, including subtle variations that can’t be seen with the naked eye, and are working in both static and dynamic domains (i.e., when the face is still or in motion). Within the project is another line of research devoted to how voice and image sync up.
Thus, if they can define down to the micro-details how human faces move and speak in reality--i.e., what’s “normal”--then any deviation therefrom, meaning any imperfect deepfake, should be detectable.
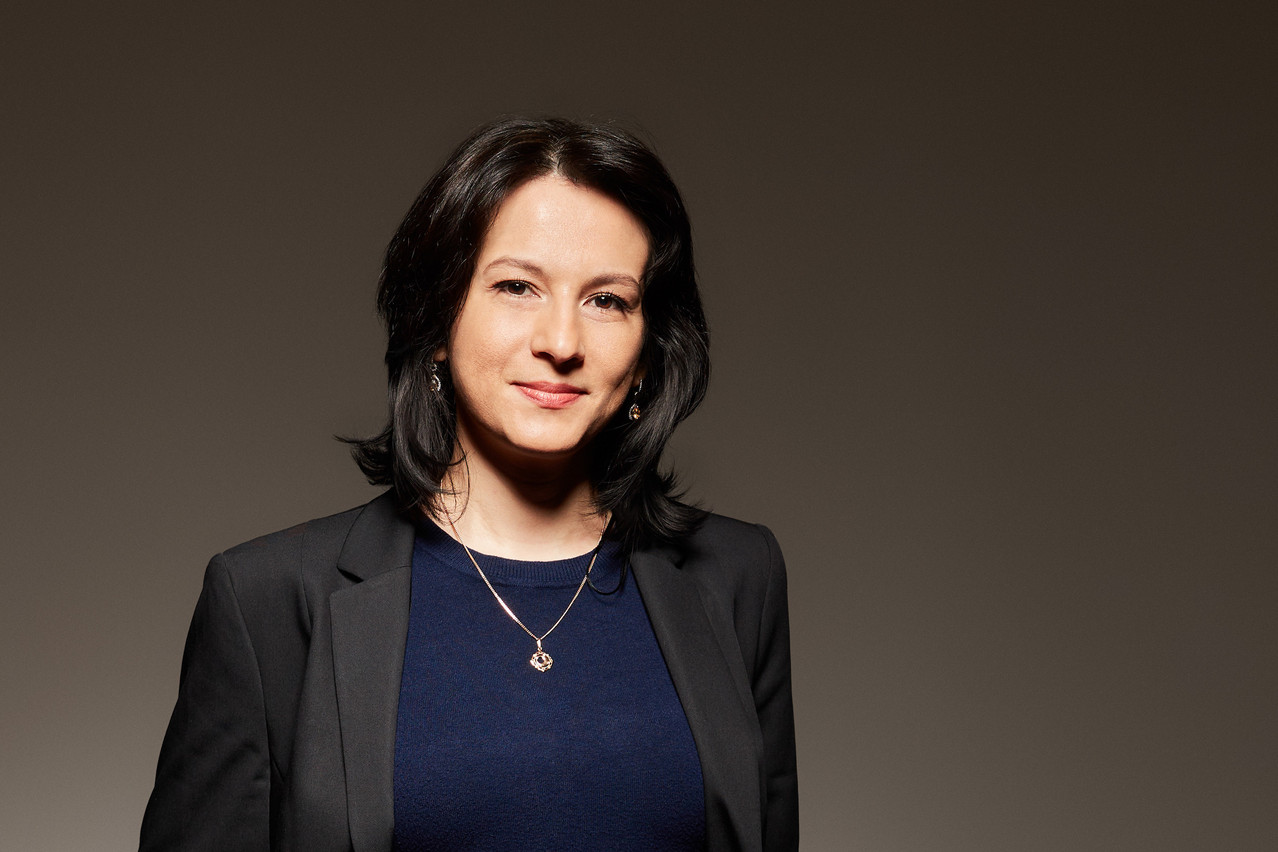
Djamila Aouada is an assistant professor, senior research scientist and head of the Computer Vision, Imaging and Machine Intelligence Research Group (CVI2) at the SnT. Photo: 101 Studios
“An achievable goal”
Deepfakes come in different types, Aouada points out, not all of which are priorities for detection. For instance, the movie industry frequently spends millions on lifelike cinematic sequences; or there is the fake Tom Cruise video that recently went viral, and which benefitted from a plethora of preexisting footage of the target to work with.
These, the researcher says, require lots of effort and resources, making them harder to detect but also a lower priority. “Our goal is more the cheap deepfake,” she says. “Those are more dangerous.”
Indeed, if any social media user can generate plausible-looking fake news with a simple plug-and-play tool, the possibilities for havoc are endless.
“I think this is an achievable goal,” she says.
Partnership with the Post
The project, funded by the FNR Bridges scheme, is being run in partnership with Post Luxembourg, which provides use cases and feedback on customer interest.
The SnT team includes five researchers: Nesryne Mejri, Kankana Roy, Enjie Ghorbel, Laura Lopez Fuentes and Djamila Aouada.